I tried out a new beta of an AI-powered wardrobe generator wardobe-ai.com. It is all it’s cracked up to be.
Let me start by saying expectations were low. How good can an app that someone kludged together and posted on Reddit be anyway? Better than I imagined. Not only was it pretty cool, I learned some things about generating a self-portrait in AI. First, I found the images generated by wardrobe-ai much more appealing than the ones generated by my earlier experiments. That may be because wardrobe-ai is using a model that is better tuned to create photorealistic images of people wearing clothing. It also could be that one needs fewer instance images to train a good model of one’s likeness.
Playing around with wardrobe-ai, I uploaded some selects from my instance images and generated a set of inferences. The results were pretty much what you would expect, although the app was very generous with my features and apparent age. I assume folks have had enough of me at this point. So, instead of using my images, I borrowed a bunch from photographer Nina on Pexels. (Thanks, Nina) In addition to being professional photographs, a woman subject provides a lot more variation in wardrobe styles.
Instance images
I pulled 13 images from Nina’s portfolio of the same model. I resized and cropped them to 1024×1024 in Photoshop. Although the app doesn’t tell you what resolution, I assumed they were using some sort of Stable Diffusion model, so it would need to be trained on square images at either 512px or 768px resolution. I figured that the app could resize easily, and I think I may have a use for these instances at some point in the future, so I made them slightly higher resolution.
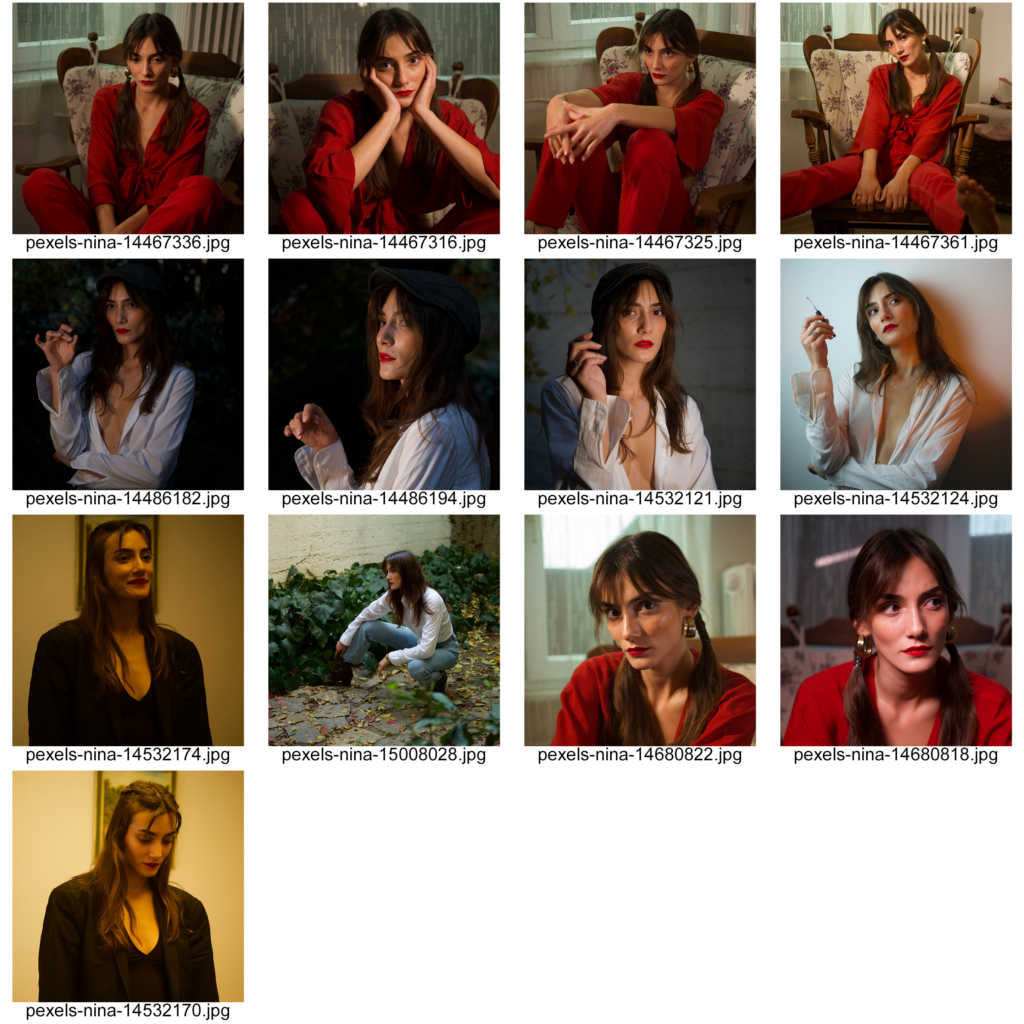
Selecting the articles of clothing for the AI-powered wardrobe
The app prompts you to select 5 of the dozen or so wardrobe articles of clothing. I chose Dress, Coat, Activewear, Suit, and Knitwear. Then I have to wait for the images to be generated. The app will send you an email when the photos are ready. Now we wait. One thing that was a little awkward is that the email letting you know that your images are ready front runs the actual app by a few minutes, so when you get the email and return to the site, the photos are yet ready.
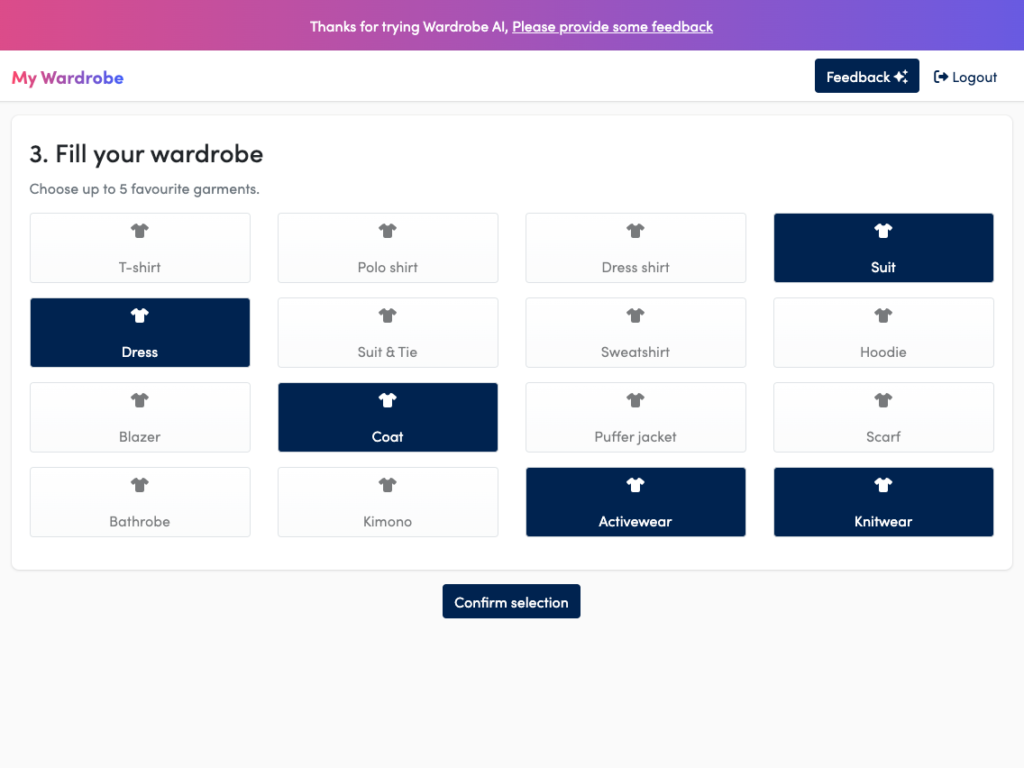
Results
I started with dresses. In the initial render, I got two images that featured sheer clothing. So, I needn’t include that in the custom prompts. I also got an image featuring corsetry. So, I can cross that off my list of prompts. As for colors, inference images in two of the articles of clothing hit on the slate blue color and had credible results some very credible results. One result image was pretty squarely in the romantic category as well. How does it do that without prompting? Ostensibly, the trends for next season don’t exist in this season, or they wouldn’t be newsworthy. I have some theories.
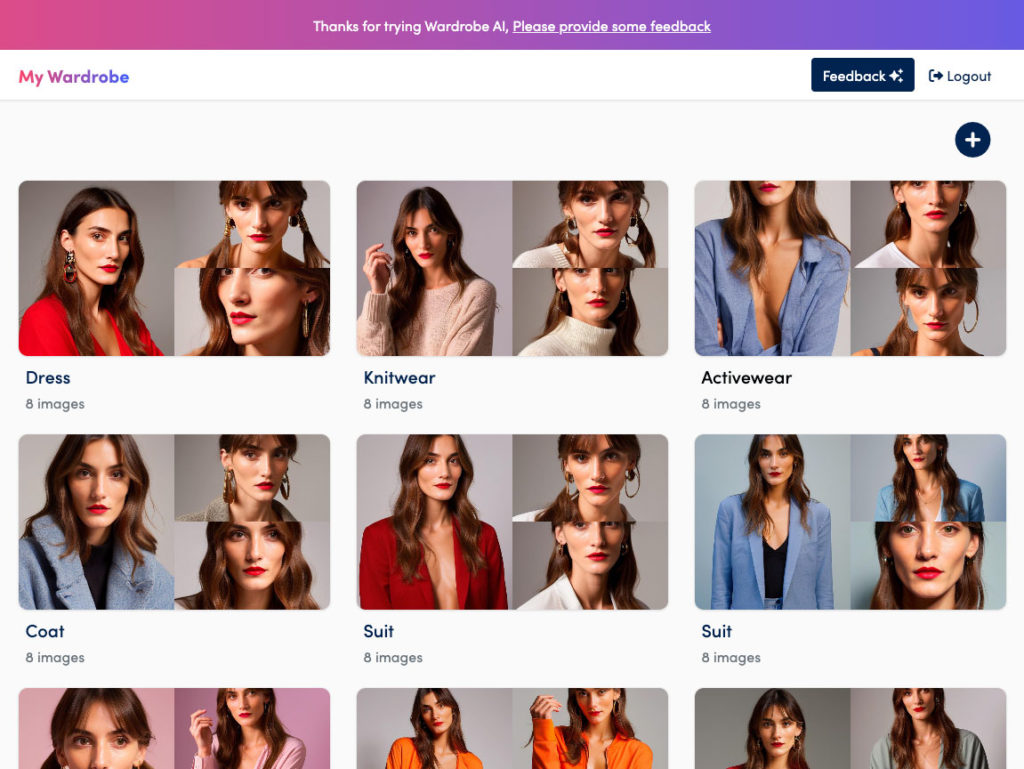
Explore products
If you click on one of the inference images, the app gives you a set of products that are visually similar to the image. You can then click through to purchase.
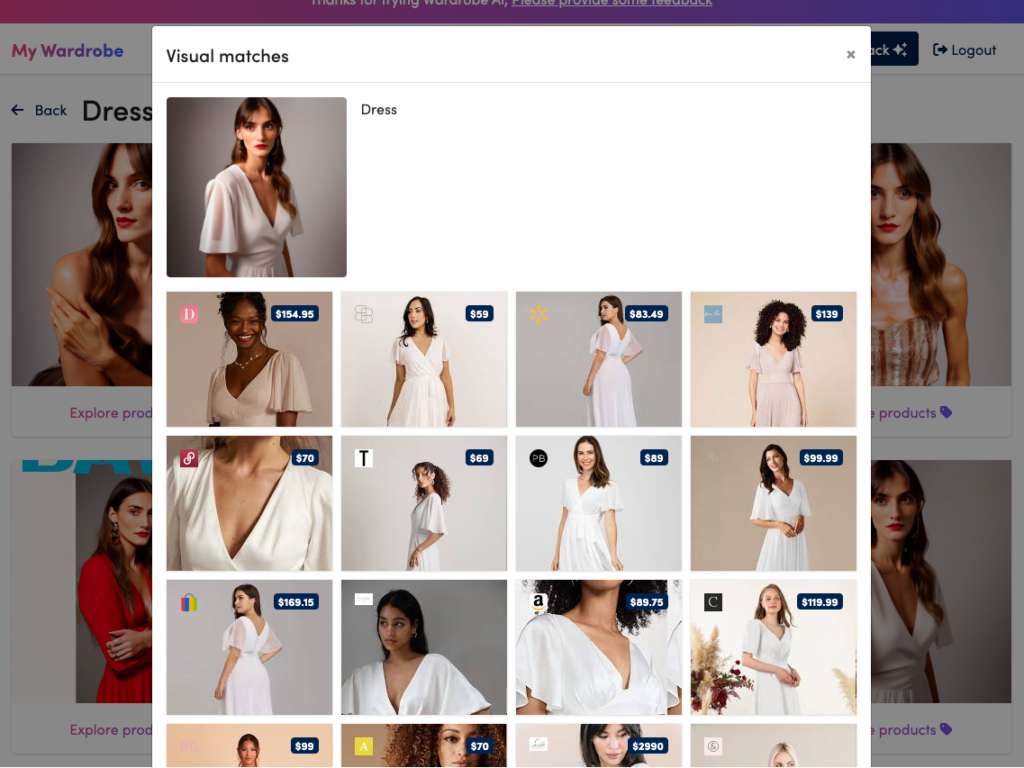
My guess of how the AI-powered wardrobe product exploration works
The app generates inference images from some canned prompts and interrogates the image using CLIP. The app then used image search and the text prompt in Google Shopping search to deliver a bunch of items for sale that meet the criteria. That I would have three trends hit on the first blush of dresses means a correlation is going on. Possibilities I considered:
- The developers are prompt hacking by prompting the model with words that describe current trends.
- Google has the trends baked into its algorithm. People are searching for 2023 trends. People are looking for clothing that fits the description. Google’s algorithm gives greater weight to trending results.
- Clothing styles don’t change that fast. These looks are always in style, so they always show up.
- The subject I trained the ai on is a model. Models tend to wear trendy looks, Ergo, the inference images returned feature the model wearing trendy looks.
Canned prompts
I noticed that the set of inferences images returned followed the same pattern. The first is always red, the second is light blue, the third is beige, and the fourth is white. So, for example, on the second row, the first is red, the second brown, the third white, and the fourth is also white.
Expanding on the stock results
The AI-powered wardrobe app gives you a bunch of looks not based on anything I can say. There is a plus button up on the top where you can request a new wardrobe article. The app gives you two input fields. One for adjectives describing the clothing, and the other for clothing description. It was not clear to me what the difference between the two is. Since the subject is not me and I do not know her likes and dislikes or knowledge of what looks good on her, I plucked my descriptors from public sources. For descriptions, I decided to research what the fashion mavens like Vogue and Trendhunters predicted to be in for Spring 2023.
2023 style trends
The looks I got were Detailed Denim, Neo-minimalism (Clean Slate), Romantic distressing, Draped dressing, Sheer layering, Maxiskirts, Red shoes, Big Bags, Pinstripe tailoring, Branded Soccer Tees, Elle prophesied Baggy Jeans, Supersized Blazers, Sheer everything, Drop waist and bubble skirts, Sequins, Leather and Balletcore, and metallic crops and corsets. Elle also predicted acid green as a dominant color. Seventeen agrees on the maxi skirts, baggy jeans, fringes, and balletcore and adds western, floral, and gothic to the mix,
Spring Colors 2023
Pantone says acid green, slate blue, light pink, orange, peach, seafoam, and leek green are in order.
Trendhunter has some more specific and marginal guesses Velvet tracksuits, Esports streetwear, British, Hairy Avant garden footwear, Artist collaborations, Y2K inspired, Unisex urban streetwear, Gaming themed luxury fashion, sustainable back-to-basics, Buddhist-inspired streetwear, Sci-Fi, Highline-inspired, That is a lot of looks from which to choose. I am most interested in the outliers. It should be reasonably easy to generate a bunch of generic looks and put them on a model. What about personalized style? Plucking some morsels from the buffet,
Custom Prompts
- Dresses: Adjs: light pink, leek green. Desc: Balletcore, ballet core, metallic, sci-fi
- Coat: Adjs.: Draping, sheer, high line-inspired, Desc.: Western, Y2K, artist collaboration
- Activewear: Adjs: Orange, peach, acid green Desc.: velvet tracksuit, balletcore, unisex, and Y2K inspired
- Suit: Adjs: Buddhist-inspired streetwear, pin-striped, neo-minimalism, metallic, British. Desc: Seafoam, Slate blue, robin’s egg blue
- In the customized menu, there isn’t a button for knitwear, so I substituted Sweatshirt for the category.
- Sweatshirt: Supersized, Esports streetwear.
Custom Results
All of the prompts generated perfectly credible looks, although nothing too far out. I’ll share the images without too much commentary.
What I want to do is discover new looks. So, I made some changes to the prompts and reran them.
Taking color out of the equation
My new strategy is to leave off the color entirely, mainly focusing on the silhouette and drape. I also tried using some syntax that is commonly available to Stable Diffusion. For the next round, I put
- Dresses: Adjs: ((metal)), sci-fi, balletcore, knitwear, fringes, new romantic,
- Activewear: Adjs: velvet tracksuit, sheer, fitted Desc.: Western, unisex, 90s, and Y2K-inspired.
- Suit: Adjs: close-fitted, tailored, pin-striped, British. Desc: neominimalist, minimalist, buttons
I had hoped these prompts would turn me on to some hidden treasures for sale. Not so. Of the three new custom ones, the Dresses delivered something closer to the prompt.
Conclusions
Wardrobe-ai is a sharp stab at making a new way to shop. The interface and the platform do a lot of heavy lifting in finding looks that work well for you. I am perhaps not the ideal market. But, having dressed myself for decades, I have a pretty good idea of what looks good on me and the styles I like. This app has a lot to offer for fast fashion merchandising against the competition.
- You can see yourself in some of the clothes you are considering purchasing.
- You don’t know what something is called, e.g., the silhouette’s name, the type of stitching, the drape, or the name of the sleeve, hem, or dart configuration. Having a visual search of inference images takes all work off your hands and hands it to the visual search engine. The AI-powered wardrobe app automates the tedious part of picking clothes.
- You find a look you like but don’t know how much it costs or what’s available at different price points. For example, will a $50 purchase deliver the same look as a $500 one? Wardobe-ai takes some of the guesswork out for you.
A ton of research has been done on recommendation engines. Just think of the number of embeddings that go into the Pinterest sort. Using Stable Diffusion as the engine may not be the best technology for the purpose. But who knows? Perhaps a general-purpose latent diffusion image generator has advantages over other methods of which we are only scratching the surface.
Bonus
I said I wouldn’t but I think you might want to see what wardobe.ai does with an ordinary person’s images. Here are mine.
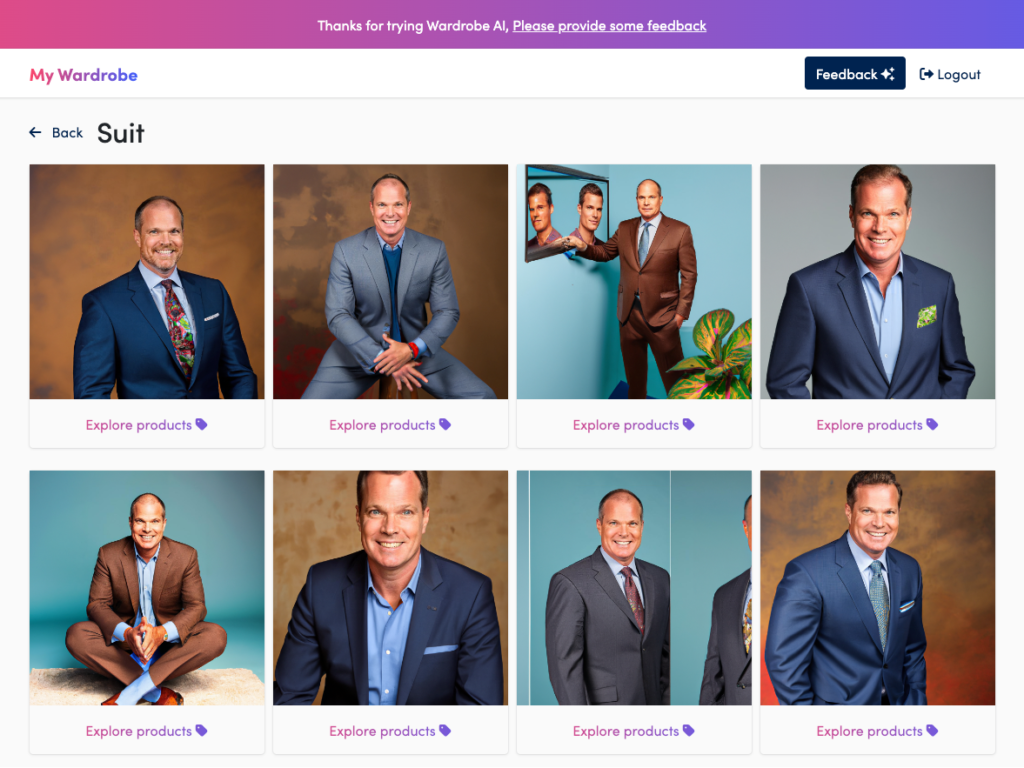